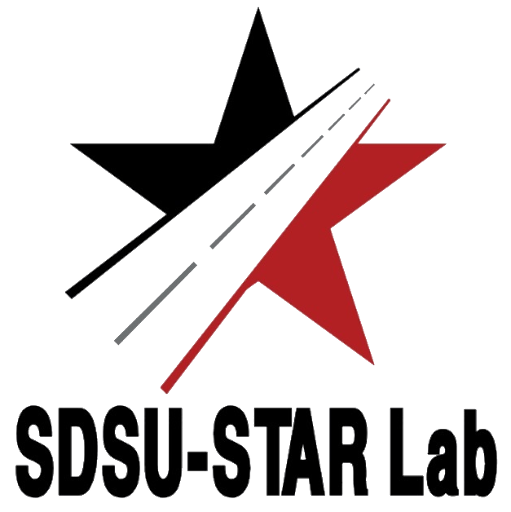
Since its foundation in 2015, the SDSU STAR lab has been involved in several research projects funded through the University Transportation Centers Program of the US Department of Transportation, National Science Foundation (NSF), and the State of California’s Senate Bill 1 (SB1). Below is a summary of these projects.
Prediction of Vehicle Trajectories at Intersections Using Inverse Reinforcement Learning
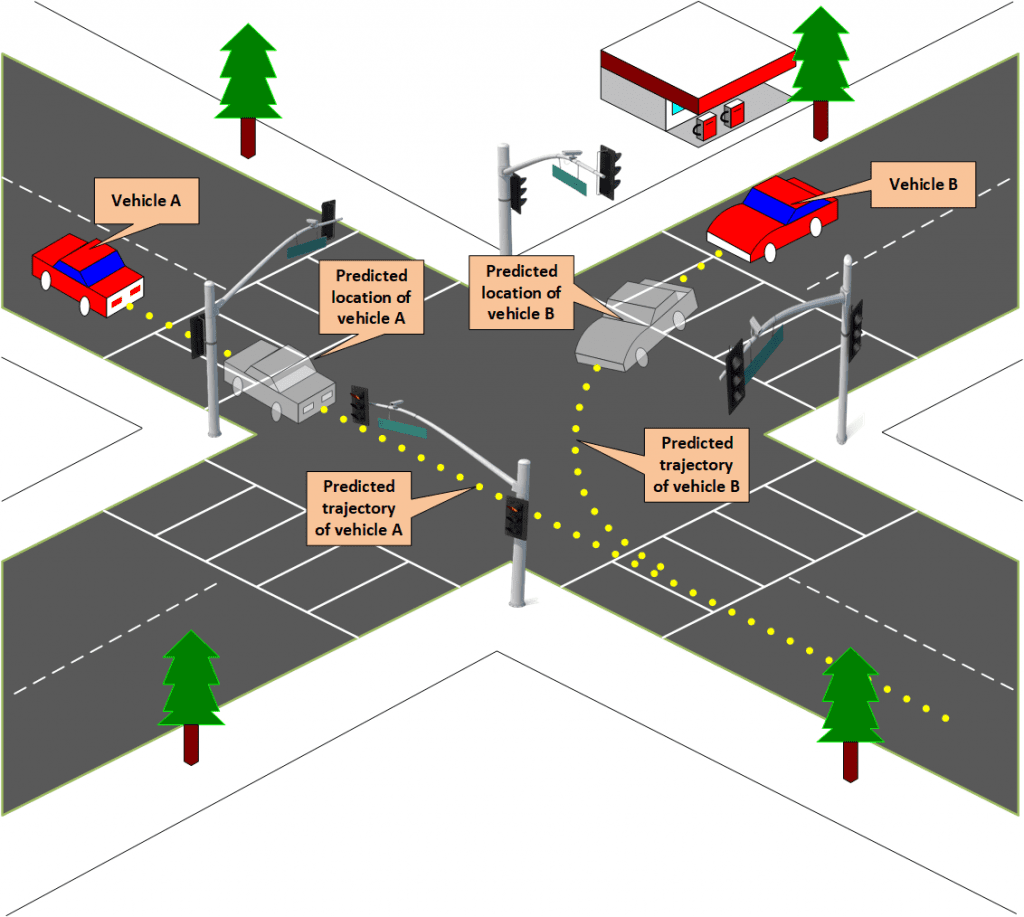
The ability to accurately predict vehicle trajectories is essential in infrastructure-based safety systems which aim to identify critical events such as near crash situations and traffic violations. In a connected environment, important information about these critical events can be communicated to road users or the infrastructure to avoid or mitigate potential crashes. Intersections require special attention in this context because they are hotspots for accidents and involve numerous and complex interactions between road users. The proposed project will investigate approaches to and methodologies for vehicle trajectory prediction at intersections, and identify current challenges and opportunities. The project will also explore the applicability of inverse reinforcement learning (IRL) in developing trajectory prediction models. IRL is a machine learning framework for Learning from Demonstration (LfD) which allows for the learning of the series of actions that dictate driver behavior from expert demonstration. Trajectory prediction, while normally posed as a prediction problem, can readily be cast as a control problem given that the problem is essentially equivalent to finding how to drive a vehicle the way a human driver would. This is crucial in enabling us to use IRL to solve it. The IRL framework makes certain assumptions about the problem to be solved that, when these assumptions hold, improve the generalizability of models that are based on. We hope that by employing this framework we will be able to build models that better capture the behavior of human drivers at intersection.
Research Investigators (PI*)
Arash Jahangiri (SDSU)*
Sahar Ghanipoor Machiani (SDSU)
Mohammad Sadegh Jazayeri (SDSU)
Sponsor
Safe-D National UTC
Office of the Assistant Secretary for Research and Technology
University Transportation Centers Program
Department of Transportation
Final Report
More Information
Safety Impact Evaluation of a Narrow Automated Vehicle-Exclusive Reversible Lane on an Existing Smart Freeway
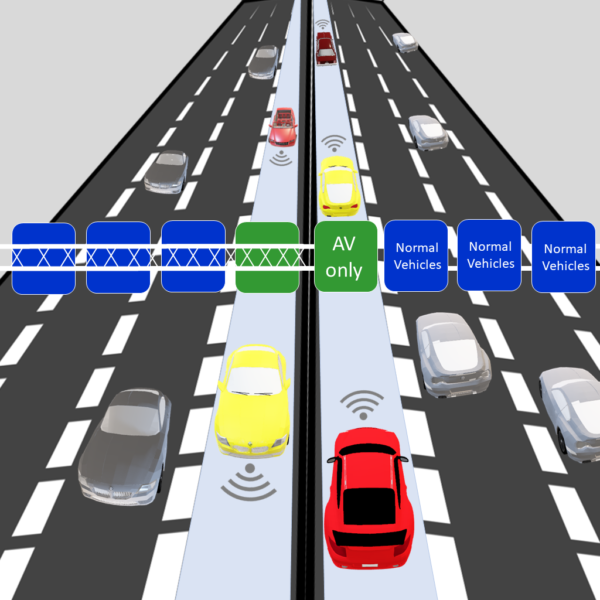
The main objective of this research is to evaluate the safety impact of an innovative infrastructure solution for safe and efficient integration of Automated Vehicle (AV) as an emerging technology into an existing transportation system. Filling the gap in the limited research on the effect of AV technology on infrastructure standards, this project will evaluate whether AVs could operate safely in a narrow lane next to regular traffic lanes on an expressway. Specifically, this study will investigate implications of adding a narrow reversible AV exclusive lane to the existing configuration of I-15 expressway in San Diego, resulting in a 9-feet AV reversible lane, and in both directions, two 12-feet lanes for HOV and FasTrak vehicles. Given the difference between the operation of AVs and human-driven vehicles and reliance of AVs on sensors as opposed to human capabilities, the question is can we provide narrower roadways with more efficient right of ways assuming AVs are more precise in lateral and longitudinal lane keeping behavior? To accomplish the goal of the project, the research team will conduct a series of research approaches including literature review, AV manufacturers product review, expert interviews, consumer questionnaire review, crash data analysis, and traffic simulation analysis. The research team will prepare practical recommendations and guidelines from the results of the study usable for practitioners and professional organizations pertaining to AV development.
Research Investigators (PI*)
Sahar Ghanipoor Machiani (SDSU)*
Arash Jahangiri (SDSU)
Walter Musial (LLG Engineers)
Shankar Ramakrishnan (LLG Engineers)
Alidad Ahmadi (LLG Engineers) Sam Amen (Caltrans)
Sponsor
Safe-D National UTC
Office of the Assistant Secretary for Research and Technology
University Transportation Centers Program
Department of Transportation
Final Report
More Information
Developing an intelligent transportation management center (ITMC) with a safety evaluation focus for smart cities
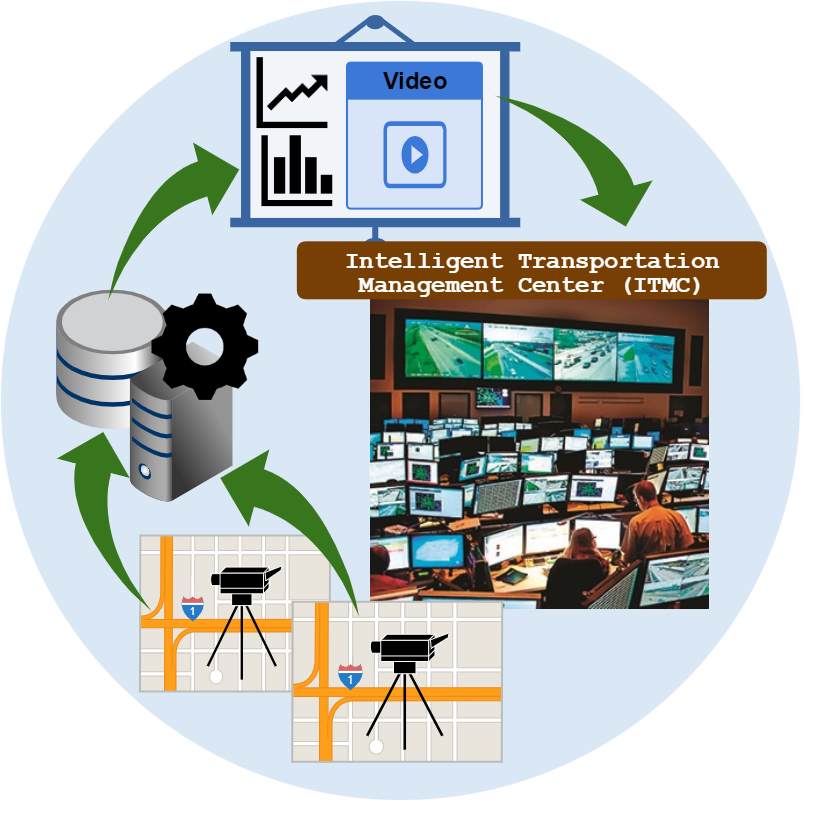
Transportation safety planning challenges in the era of smart cities entail understanding safety impacts from disruptive technologies, measuring the effectiveness of safety countermeasures, proactively identifying high crash risk locations, etc. Recent advancement in communication technologies and big data analytics enables us to deal with these challenges in a computationally efficient way. Traditional transportation management centers (TMCs) have limited capability to utilize large amounts of data to properly evaluate transportation safety. The goal of this project is to develop an intelligent transportation management center (ITMC) that adopts automated video data analysis to evaluate safety. The proposed ITMC demonstrates how intelligent transportation systems (ITS) technologies and big data analytics can be utilized to proactively assess transportation safety at signalized intersections. Conventional methods of traffic safety risk assessment at signalized intersections, measured by number of roadway crashes per unit of exposure, would require a long observation time as crashes are rare events. The proposed ITMC adopts safety surrogate measures (SSMs) to identify near crash situations that can be applied in proactive risk calculations.
Research Investigators (PI*)
Arash Jahangiri (SDSU)*
Christopher P. Paolini (SDSU)
Sahar Ghanipoor Machiani (SDSU)
Alireza Darzian Rostami (SDSU)
Zac Doerzaph (VTTI)
Mike Mollenhauer (VTTI)
Sponsor
Safe-D National UTC
Office of the Assistant Secretary for Research and Technology
University Transportation Centers Program
Department of Transportation
Final Report
More Information
Evaluation of Driving Ability after Mild Traumatic Brain Injury
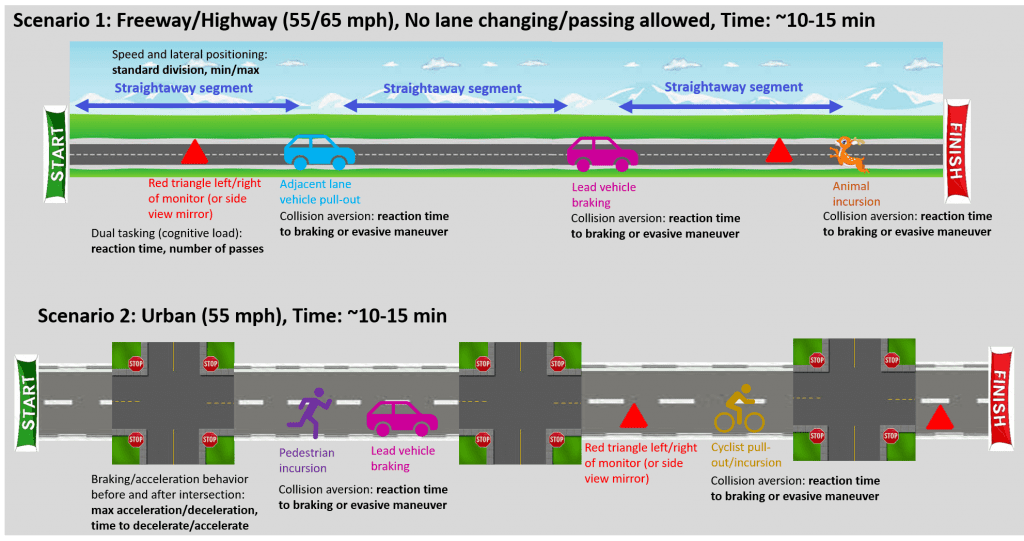
Due to the limited clinical evidence supporting driving fitness post-mild traumatic brain injury (TBI), this study aims to investigate driving performance in a multi-week follow up timeline with patients after mild TBI (mTBI) by comparing selected outcomes of patients with and without mTBI. Trauma patients will be consented through post-resuscitation room and study intervention will take place at San Diego State University. Neuropsychological assessments including the Rivermead Post-Concussion Symptoms Questionnaire (RPQ) and the Trail Making Test (TMT) will be collected before each driving simulator session. A Simulator Sickness Questionnaire (SSQ) will be administered after the adaptation drive to evaluate the participant’s reaction to the simulator drive. Speed, perception-reaction times, dual-tasking or cognitive load, rates of acceleration, and car following behavior will be collected in a 30-minute driving simulation. The results of the study may contribute to future concussion/mTBI evaluation and management guidelines on driving ability and inform recommendations regarding when patients can return back to driving after mTBI.
Research Investigators (PI*)
Sahar Ghanipoor Machiani (SDSU)*
Arash Jahangiri (SDSU)
Walter Biffl (Scripps)
Sponsor
Scripps Health
Final Report
More Information
Big Data Visualization and Spatiotemporal Modeling of Aggressive Driving
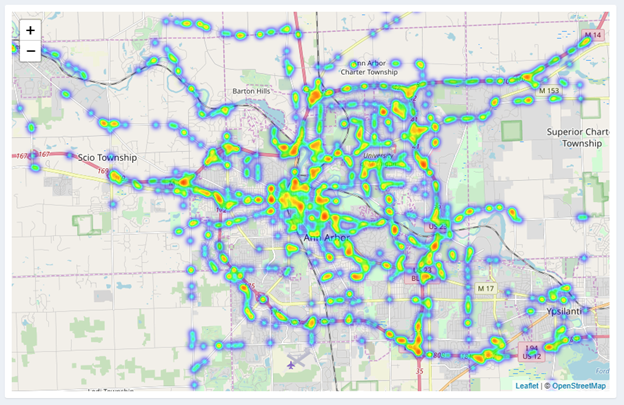
More than half of fatal traffic crashes occur due to aggressive driving according to AAA (American Automobile Association) Foundation for Traffic Safety. Ubiquitous technology has made it possible to monitor driver behavior at a high frequency for a long period of time. This provides an opportunity for researchers to investigate risky driving behavior at a large scale. This project aims to develop a big data analytics framework and visualization tool to conduct spatiotemporal modeling and classify and visualize aggressive driving behavior using data from emerging technology. As an essential safety planning tool in the era of big data, this framework/tool can be used to identify where and when aggressive driving occurs. The Safety Pilot Model Deployment (SPMD) data, one of large transportation datasets available via the FHWA Research Data Exchange (RDE) program will be used as a big data example. A classification model will be developed to discriminate aggressive driving from normal driving using kinetic and environmental GIS data. Web-based data visualization tools will also be developed to identify the spatial and temporal distribution of aggressive driving. Furthermore, data visualization in a simulated real-time scenario will be conducted to produce insights from future transportation data considering the anticipated widespread adoption of autonomous and connected vehicle technology.
Research Investigators (PI*)
Arash Jahangiri (SDSU)*
Sahar Ghanipoor Machiani (SDSU)
Ming-Hsiang Tsou (SDSU)
Atsushi Nara (SDSU)
Charles Marks (SDSU)
Leslie Harwood (VTTI/VT)
Sponsor
Safe-D National UTC
Office of the Assistant Secretary for Research and Technology
University Transportation Centers Program
Department of Transportation
Final Report
More Information
Impact of Regular and Narrow AV-Exclusive Lanes on Manual Driver Behavior
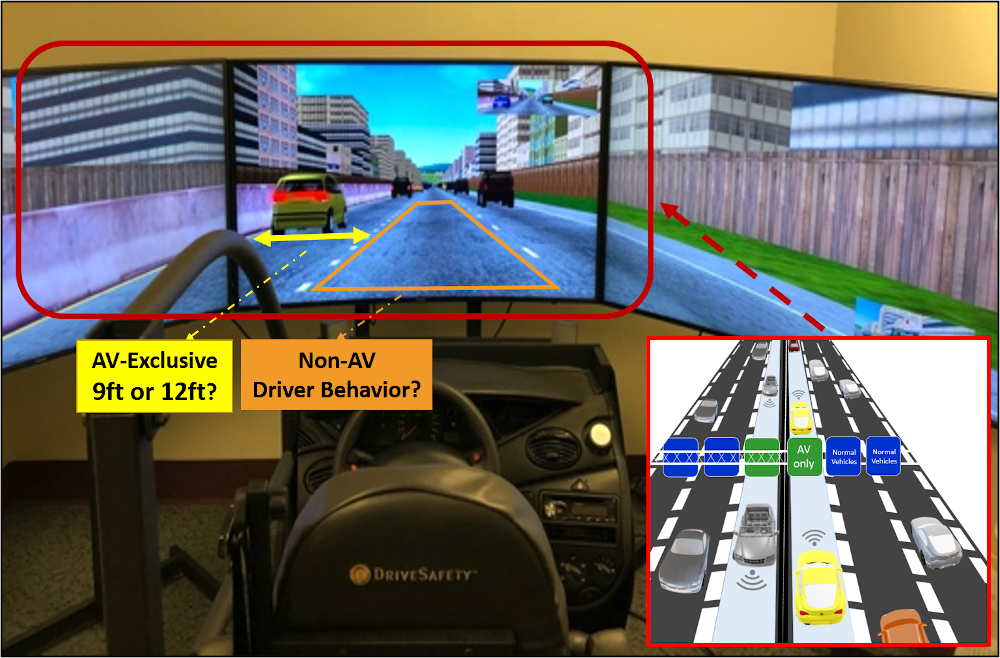
This study attempts to answer the question of how a narrow (9-ft) lane dedicated to Automated Vehicles (AVs) would affect the behavior of drivers in the adjacent lane to the right. To this end, a custom driving simulator environment was designed mimicking the Interstate 15 smart corridor in San Diego. A group of participants was assigned to drive next to the simulated 9-ft narrow lane while a control group was assigned to drive next to a regular 12-ft AV lane. Driver behavior was analyzed by measuring the mean lane position, mean speed, and mental effort (self-reported/subjective measure). In addition to AV lane width, the experimental design took into consideration AV headway, gender, and right lane traffic to investigate possible interaction effects. The results showed no significant differences in the speed and mental effort of drivers while indicating significant differences in lane positioning. Although the overall effect of AV lane width was not significant, there were some significant interaction effects between lane width and other factors (i.e., driver gender and presence of traffic on the next regular lane to the right). Across all the significant interactions, there was no case in which those factors stayed constant while AV lane width changed between the groups, indicating that the significant difference stemmed from the other factors rather than the lane width. However, the trend observed was that drivers driving next to the 12-ft lane had better lane centering compared to the 9ft lane. The analysis also showed that while in general female drivers tended to drive further away from the 9-ft lane and performed worse in terms of lane centering, they performed better than male drivers when right-lane traffic was present. This study contributes to understanding the behavioral impacts of infrastructure adaptation to AVs on non-AV drivers.
Research Investigators (PI*)
Sahar Ghanipoor Machiani (SDSU)*
Aryan Sohrabi (SDSU)
Arash Jahangiri (SDSU)
Sponsor
State of California SB1 2017/2018
Trustees of the California State University
Sponsored Programs Administration
Final Report
More Information
Developing a Computer Vision-Based Decision Support System for Intersection Safety Monitoring and Assessment of Vulnerable Road Users
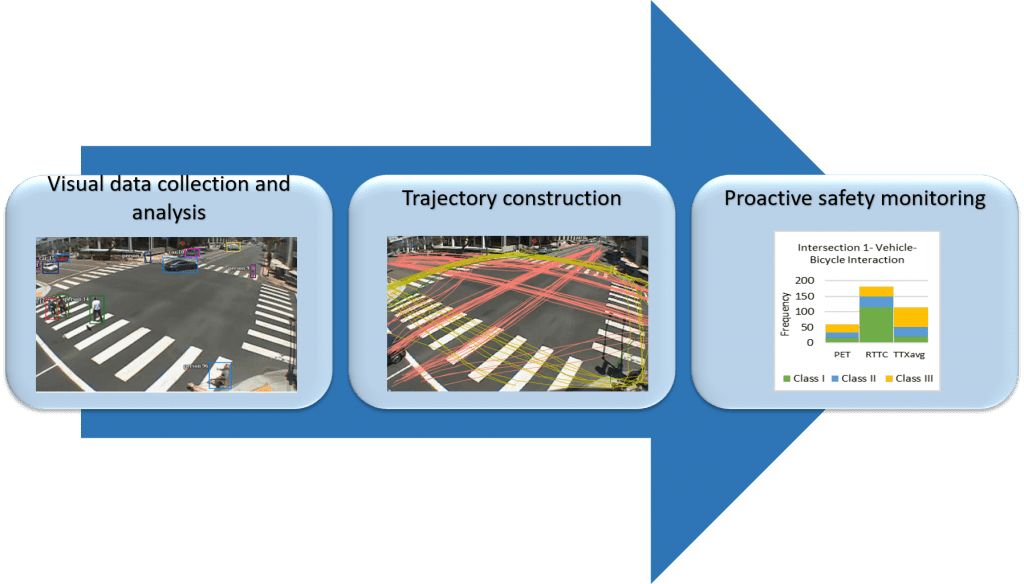
Vision-based trajectory analysis of road users enables identification of near-crash situations and proactive safety monitoring. The two most widely used sur-rogate safety measures (SSMs), time-to-collision (TTC) and post-encroachment time (PET)—and a recent variant form of TTC, relative time-to-collision (RTTC)—were investigated using real-world video data collected at ten signalized intersections in the city of San Diego, California. The performance of these SSMs was compared for the purpose of evaluating pedestrian and bicyclist safety. Prediction of potential trajectory intersection points was performed to calculate TTC for every interacting object, and the average of TTC for every two objects in critical situations was calculated. PET values were estimated by observing potential intersection points, and frequencies of events were estimated in three critical levels. Although RTTC provided useful information regarding the relative distance between objects in time, it was found that in certain conditions where objects are far from each other, the interaction between the objects was incorrectly flagged as critical based on a small RTTC. Comparison of PET, TTC, and RTTC for different critical classes also showed that several interactions were identified as critical using one SSM but not critical using a different SSM. These findings suggest that safety evaluations should not solely rely on a single SSM, and instead a combination of different SSMs should be considered to ensure the reliability of evaluations. Video data analysis was conducted to develop object detection and tracking models for automatic identification of vehicles, bicycles, and pedestrians. Outcomes of machine vision models were employed along with SSMs to build a decision support system for safety assessment of vulnerable road users at signalized intersections. Promising results from the decision support system showed that automated safety evaluations can be performed to proactively identify critical events. It also showed challenges as well as future directions to enhance the performance of the system.
Research Investigators (PI*)
Arash Jahangiri (SDSU)*
Anagha Katthe (SDSU)
Aryan Sohrabi (SDSU)
Xiaobai Liu (SDSU)
Shashank Pulagam (SDSU)
Vahid Balali (CSULB)
Sahar Ghanipoor Machiani (SDSU)
Sponsor
State of California SB1 2017/2018
Trustees of the California State University
Sponsored Programs Administration
Final Report
More Information
Data Mining to Improve Planning for Pedestrian and Bicyclist Safety
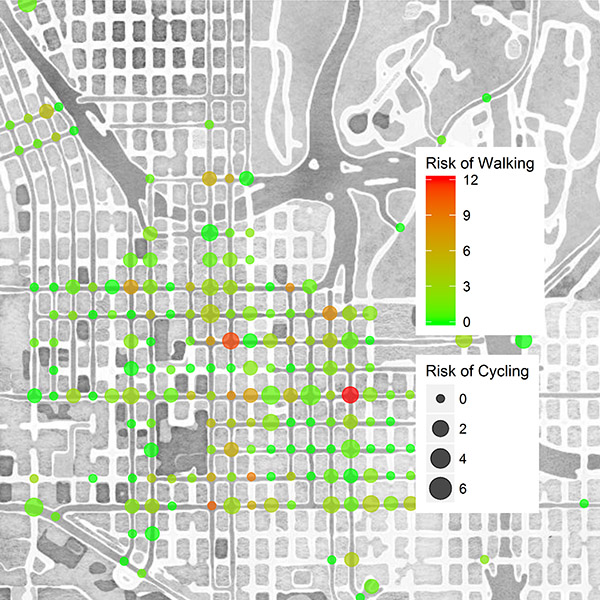
Between 2009 and 2016, the number of pedestrian and bicyclist fatalities saw a marked trend upward. Taken together, the overall percentage of pedestrian and bicycle crashes now accounts for 18% of total roadway fatalities, up from 13% only a decade ago. Technological advancements in transportation have created unique opportunities to explore and investigate new sources of data for the purpose of improving safety planning. This study investigated data from multiple sources, including automated pedestrian and bicycle counters, video cameras, crash databases, and GPS/mobile applications, to inform bicycle and pedestrian safety improvements. Data mining techniques, a new sampling strategy, and automated video processing methods were adopted to demonstrate a holistic approach that can be applied to identify facilities with highest need of improvement. To estimate pedestrian and bicyclist counts at intersections, exposure models were developed incorporating explanatory variables from a broad spectrum of data sources. Intersection-related crashes and estimated exposure were used to quantify risk, enabling identification of high-risk signalized intersections for walking and bicycling. The modeling framework and data sources used in this study will be beneficial in conducting future analyses for other facility types, such as roadway segments, and also at more aggregate levels, such as traffic analysis zones.
Research Investigators (PI*)
Arash Jahangiri (SDSU)*
Sherry Ryan (SDSU)
Bruce Appleyard (SDSU)
Ipek N. Sener (TTI/TAMU)
Shawn Turner (TTI/TAMU) Justin Owens (VTTI/VT)
Sponsor
Safe-D National UTC
Office of the Assistant Secretary for Research and Technology
University Transportation Centers Program
Department of Transportation
Final Report
More Information
Integrated Wildfire Evacuation Decision Support System (IWEDSS) with Stage-based Robust Evacuation Model, Social Perception Analysis, and Dynamic Population Estimation
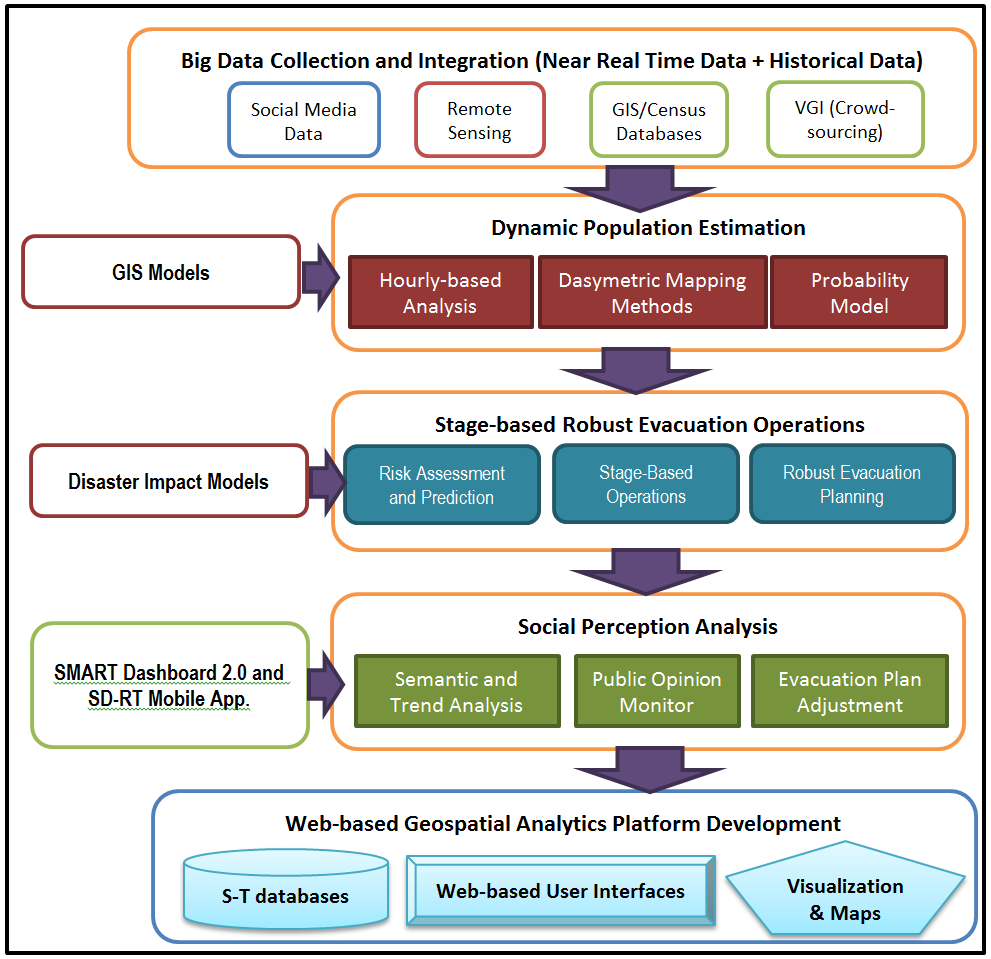
The research will help emergency response agencies better understand public perceptions and needs during disaster events, and create more effective evacuation plans for local communities. This project will integrate multiple data sources-including social media, census survey, geographic information systems (GIS) data layers, volunteer suggestions, and remote sensing data-to develop an integrated wildfire evacuation decision support system (IWEDSS) for the County of San Diego as a demonstration prototype system. IWEDSS will consist of four core modules: dynamic population estimation, stage-based robust evacuation models, social perception analysis, and a web-based geospatial analytics platform. It will offer scientifically-based and data-driven analytic tools for evacuation planers, resource managers, and decision makers to support efficient and effective decision-making activities that can reduce the evacuation time and potential number of injuries and deaths. The research team will collaborate with staff from the Office of Emergency Services (OES) of San Diego County, the San Diego/Imperial Counties Chapter of the American Red Cross, and 2-1-1 San Diego to develop IWEDSS together.
Research Investigators (PI*)
Ming-Hsiang Tsou (SDSU)*
Sahar Ghanipoor Machiani (SDSU)
Atsushi Nara (SDSU)
Terry Yang (Uni of Utah)
Sponsor
National Science Foundation (NSF)
Final Report
More Information
A Holistic Work Zone Safety Alert System through Automated Video and Smartphone Sensor Data Analysis
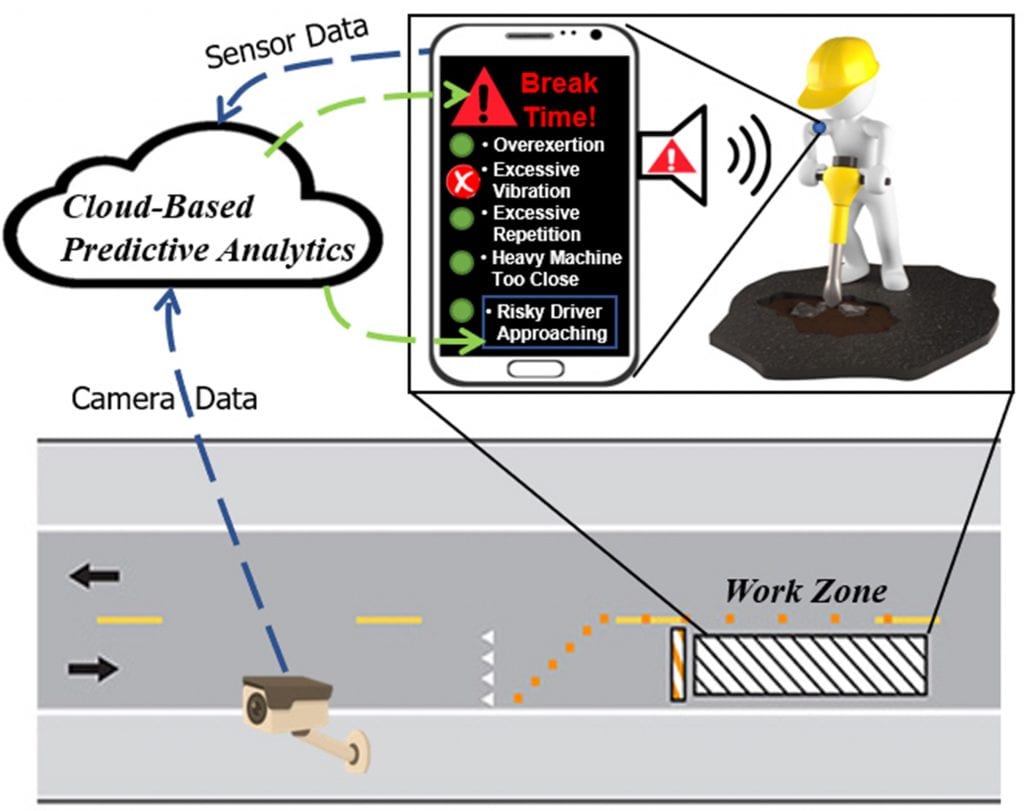
This project is inspired by major gaps identified in the literature pertaining to the work zone safety monitoring systems that leverage advanced technologies for tracking workers, identifying hazardous situations, and alerting individuals in danger. The existing systems have two key shortcomings. First, they either target safety hazards external to the work zone (e.g., only vehicular accidents) or workers’ state internally and related to their physical/physiological state (e.g., only human-factor ergonomics or fatigue). Second, previous work fails to address the lack of trust as an adoption barrier of such technologically advanced systems. This project presents a hybrid approach in which visual- and wearable-sensor data are used for safety monitoring and alert generation to offer a practical mitigation strategy to both external and internal safety risks. It leverages smartphones as a pervasive and standalone resource for collecting data and communicating safety-related instructions to workers. The project also uses information systems and behavioral science theories (i.e., technology acceptance model and Diffusion of Innovation theory) to build end-users trust toward scalable adoption of the developed systems.
Research Investigators (PI*)
Reza Akhavian (SDSU)*
Arash Jahangiri (SDSU)
Sponsor
Safe-D National UTC
Office of the Assistant Secretary for Research and Technology
University Transportation Centers Program
Department of Transportation
Final Report
More Information
Evaluation of transportation safety against flooding in disadvantaged communities
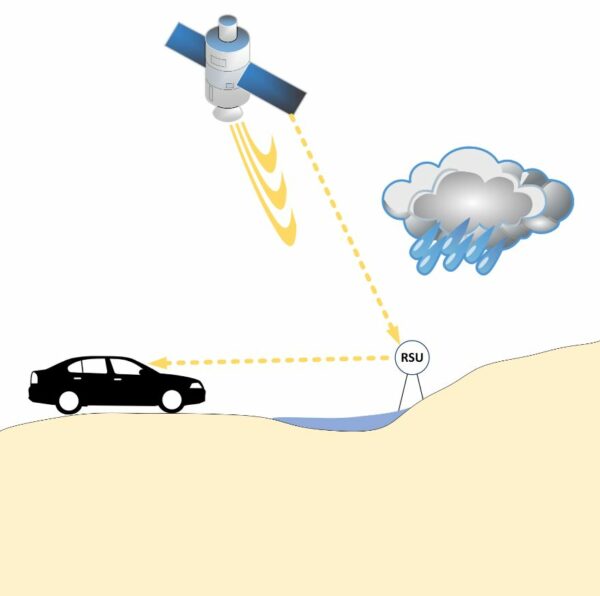
The goal of the proposed project is to systematically extract traffic safety information from multiple complex sources of flood monitoring such as remote sensing technologies, flow gages, and weather stations, which can support informed planning for transportation safety against flooding in future smart cities. Flooding poses a significant hazard to the moving vehicles and causes traffic disruption by placing water flow in the transportation network, resulting in sweeping vehicles away, injuries and loss of life of passengers. While different methods to continuously monitor flooding are available in the field of flood management, the collected data is too complex to directly offer relevant information for transportation safety. As a result, the application of flood monitoring in Connected Vehicles has been limited to weather information, which does not directly relate to transportation safety. To fill this gap, the research team will employ Big Data Analytics to extract transportation safety information from multiple monitoring sources, each of which continuously collect data in a fine temporal scale. This approach, as an example application, will provide a decision support system to identify and prioritize candidate locations for future installation of roadside units. To facilitate visualization of their findings for an enhanced technology transfer, the team will define metrics following the risk assessment of flooding in transportation network. Consequently, the team will develop regional “heat maps” to visualize such metrics across large geographic scales, including communities that are disadvantaged.
Research Investigators (PI*)
Hassan Tavakol (SDSU)*
Sahar Ghanipoor Machiani (SDSU)
Vincent O’Hara-Rhi (SDSU)
Sponsor
Safe-D National UTC
Office of the Assistant Secretary for Research and Technology
University Transportation Centers Program
Department of Transportation
Final Report
More Information